Our lab employs multimodal neuroscience approaches and studies attention, faces, and social behavior in both healthy and neurological populations. Example research projects include, but are not limited to, the following:
Investigating the neural basis for goal-directed visual attention
Using single-unit recording with concurrent eye tracking, we identified a group of “target neurons” in the human medial temporal lobe, which specifically differentiated fixations on search targets vs. distractors. This target response was predominantly determined by top-down attention and independent of bottom-up factors such as visual category selectivity or the format of search goals. In contrast, visual category selectivity was encoded by a largely separate group of neurons and was unaffected by task demands. Our data thus show that neurons in the human MTL not only encode bottom-up object categories but also top-down goal signals with behavioral relevance.
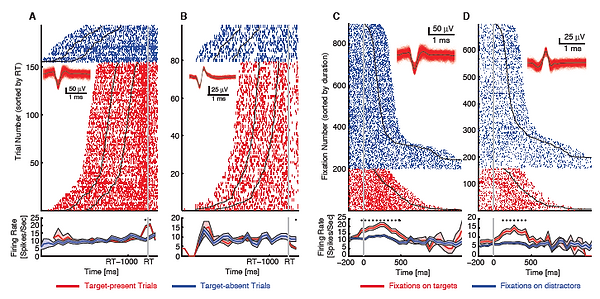
Figure legend: Example MTL target neurons. Each raster (upper) and PSTH (lower) is shown with color coding as indicated. (A-B) Trial-aligned examples (aligned at button press). Asterisk indicates a significant difference between the response to target-present trials and target-absent trials in that bin (Bonferroni-corrected). Shaded area denotes ±SEM across trials. (C-D) Fixation-aligned examples (aligned at fixation onset). Asterisk indicates a significant difference between the response to fixations on targets and fixations on distractors in that bin. Shaded area denotes ±SEM across fixations.
There are several ensuing questions: what is the nature of these target responses? Do they encode working memory, reward, and/or attention? What is the relationship between these target signals and the goal-directed signals in the other brain areas? We are conducting several control tasks and using spike-field coherence to analyze connectivity. Since the stimuli were designed for autism research (Wang et al., Neuropsychologia, 2014), they also contain many autism-related special-interest objects, and we can further test whether patients with autism have (1) altered target saliency and/or (2) different category tuning, and (3) whether category tuning will modulate target response and/or response to target-congruent search items. This will tell us whether the amygdala represents any stimulus saliency, and whether what is salient differs in people with autism.
Investigating the neural basis for saliency and memory using natural scene stimuli
One key research project in our lab is to use complex natural scene images to study saliency, attention, learning, and memory. In our previous studies, we annotated more than 5,000 objects in 700 well characterized images and recorded eye movements when participants looked at these images (Wang et al., Neuron, 2015). We will extend the same task to single-neuron recordings to investigate the neural correlates of saliency. We will also add three important components to this free viewing task: (1) we will repeat the images once or twice to explore a repetition effect (c.f. Jutras et al., PNAS, 2013), (2) we will ask neurosurgical patients to memorize the images during the first session (learning session) and test memory on the next day (recognition session) to explore a memory effect, and (3) we will explore memory encoding with overnight recording. Moreover, to probe the neural basis for altered saliency representation in autism (c.f. Wang et al., Neuron, 2015), we will analyze whether neurons are tuned to different saliency values and whether AQ/SRS scores correlate with the firing rate of fixations on semantic attributes such as faces.
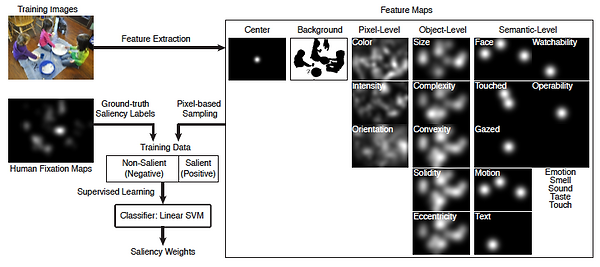
Figure legend: An overview of the computational saliency model. We applied a linear SVM classifier to evaluate the contribution of five general factors in gaze allocation: the image center, the grouped pixel-level, object-level and semantic-level features, and the background. Feature maps were extracted from the input images and included the three levels of features (pixel-, object-, and semantic-level) together with the image center and the background. We applied a pixel-based random sampling to collect the training data and trained on the ground-truth actual fixation data. The SVM classifier output were the saliency weights, which represented the relative importance of each feature in predicting gaze allocation.
Investigating the neural basis for face processing using computational face models
The human amygdala has long been associated with a key role in processing faces. For example, our own research has shown that single neurons in the human amygdala encode subjective judgment of facial emotions, rather than simply their stimulus features (Wang et al., PNAS, 2014). Using a unique combination of single-unit recording, fMRI and patients with focal amygdala lesions, we found that the human amygdala parametrically encodes the intensity of specific facial emotions and their categorical ambiguity (Wang et al., Nat Commun, 2017). Although there is a large literature on face perception, most of the studies in this tradition focus on recognition of faces and recognition of expressions of emotions, or a particular social attribute such as trustworthiness. However, it remains largely unclear how the brain represents and evaluates the faces in general. Reducing a high-level social attribution like trustworthiness to the physical description of the face is far from trivial, because the space of possible variables driving social perception is infinitely large, thus posing an insurmountable hurdle for conventional approaches. To solve this issue, we are developing a data-driven computational face model to identify and visualize the stimulus variation in faces that drive neural responses in various brain areas, including the amygdala, hippocampus and fusiform gyrus.
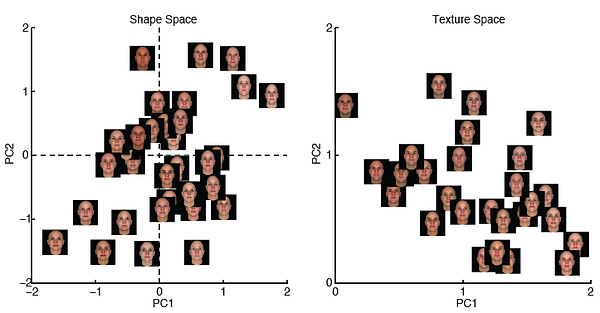
Figure legend: Computer generated random faces shown in the shape space (left) and texture space (right).
Our face model will feature the following advantages: (1) it is free of any priors and not restricted to model a particular social attribute. (2) It is independent of explicit judgments, mimicking the real-world scenario when people form instantaneous impressions of others. (3) It is able to integrate responses from different measurements, such as behavioral judgment, eye movement, fMRI BOLD signal, intracranial EEG, and single-unit response. Because all measures can be transformed to the same metric (feature weights), we can directly analyze the similarity and link the computations between measurements. (4) Related to (3), our model can not only analyze the temporal dynamics of face representation, but also the spatial distribution of face representation. It can combine the strength (i.e., spatial and temporal resolutions) from different approaches, and the multimodal approaches allow us to understand face evaluation from different angles. Therefore, our data-driven computational framework will represent an essential first step towards understanding the neural basis of complex human social perception.
Investigating the psychological and neural bases underlying altered saliency representation in autism
People with autism spectrum disorder (ASD) are characterized by impairments in social and communicative behavior and a restricted range of interests and behaviors (DSM-5, 2013). An overarching hypothesis is that the brains of people with ASD have abnormal representations of what is salient, with consequences for attention that are reflected in eye movements, learning, and behavior. In our prior studies, we have shown that people with autism have atypical face processing and atypical social attention. On the one hand, people with autism show reduced specificity in emotion judgment (Wang and Adolphs, Neuropsychologia, 2017), which might be due to abnormal amygdala responses to eyes vs. mouth (Rutishauser et al., Neuron, 2013). On the other hand, people with autism show atypical bottom-up attention to social stimuli during free viewing of natural scene images (Wang et al., Neuron, 2015), impaired top-down attention to social targets during visual search (Wang et al., Neuropsychologia, 2014), and abnormal photos taken for other people, a combination of both bottom-up and top-down attention (Wang et al., Curr Biol, 2016). However, the underlying mechanisms for these profound social dysfunctions in autism remain largely unknown.
Our lab focuses on two core social dysfunctions in autism: impaired face processing and impaired visual attention. The central hypothesis is that people with autism have altered saliency representation compared to controls. A key neural structure hypothesized to underlie the deficits in autism is the amygdala. Using a powerful combination of neuroscience techniques including single-neuron recording, functional magnetic resonance imaging (fMRI), and amygdala lesion patients, which converged on the amygdala, as well as high-resolution eye tracking and computational modeling approaches suitable for big data analysis, we investigate the following questions: (1) What are the neural underpinnings for abnormal fixations onto faces in autism? (2) What are the individual differences in autism when viewing natural scenes and what are the underlying psychological factors? Can we do large-population screening of autism given such individual differences? (3) What are the neural mechanisms for goal-directed social attention and is there a difference in people with autism?